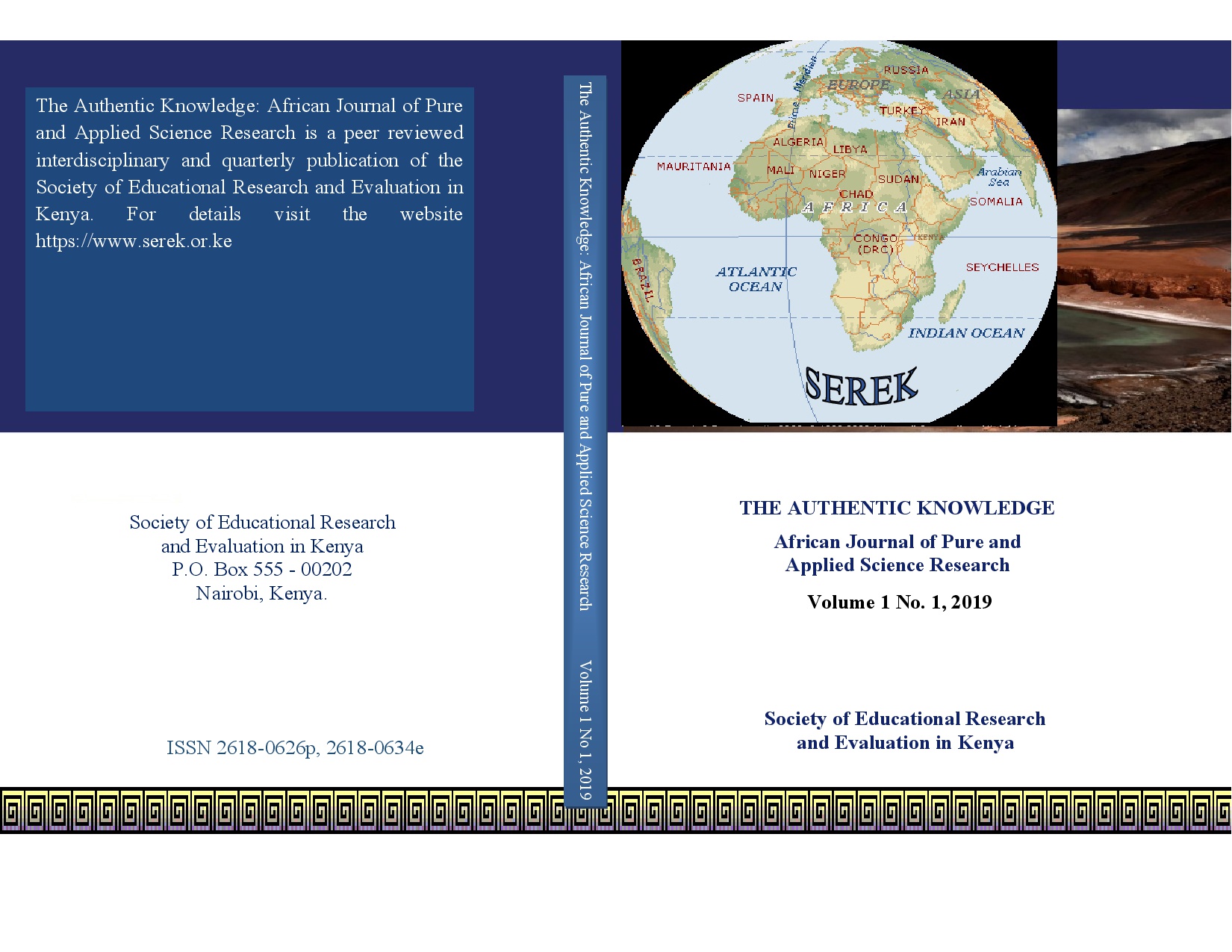
Predictions of Indigenous Chicken Phenotypes from Genotypes: Comparison Between Machine Learning and conventional Linear Models
Abstract
Genomic selection is a new breeding strategy which is rapidly becoming the method of choice of selection. It is useful in predicting the phenotypes of quantitative traits based on genome-wide markers of genotypes using conventional predictive models such as ridge regression best linear unbiased prediction model (BLUP). However, these conventional predictive models are faced with a statistical challenge related to the high dimensionality of marker data, inter and intra-allelic interactions and typically make strong assumptions. Machine learning models can be used as an alternative in the prediction of phenotypes due to their ability to address this challenges. Therefore, the aim of this study was to compare the predictive ability of machine learning using deep convolutional neural network (DeepGS), conventional neural network (Artificial neural network), conventional statistical predictive model ridge regression best linear unbiased (RR-BLUP) model and combination of DeepGS and RR-BLUP( Ensemble model) in predicting body weight (BW) of indigenous chicken based on genome-wide markers. The pearson correlation coefficient (PCC) results from this study for the four models were 0.891,0.889, 0.892 and 0.812 for DeepGS, RR-BLUP, Ensemble and ANN. This showed that DeepGS did not yield significant difference (p>0.05) from the other models, therefore, it can be used in complement to the commonly used conventional models. For individuals with higher phenotypic values, the PCC results showed a drastic decrease in the performance of DeepGS, rrBLUP, Ensemble and ANN from 0.891, 0.889, 0.892, 0.845 to 0.315, 0.466, 0.342, 0.518 respectively. Therefore, more effort should be put on individuals with higher phenotypic values.
Keywords: Genomic prediction, Machine learning, DeepGS, Artificial neural network
Full Text:
PDFReferences
Bellot, P., de los Campos, G., & Perez-Enciso, M. (2018). Can Deep Learning Improve Genomic Prediction of Complex Human Traits? Genetics, 210(3), 809-819.
Besic, L., Muhovic, I., Asic, A., Catic, A., Gurbeta, L., & Badnjevic, A. Application of neural networks to the prediction of a phenotypic trait of pacific lampreys based on single nucleotide polymorphism (SNP) genetic markers. Biomedical Research and Clinical Practice, 2(5), 1-7.
Calus, M.P.L., Berry, D.P., Banos, G., de Haas, Y., Veerkamp, R.F., (2013). Genomic selection: the option for new robustness traits. Advances in Animal Biosciences. 4:3, 618–625
Favaro, L., Briefer, E. F., & McElligot, A. G. (2014). Artifcial Neural Network approach for revealing individuality, group membership and age information in goat kid contact calls. Acta Acustica United with Acustica, 100(4), 782-789.
Groenen, M. A., Megens, H. J., Zare, Y., Warren, W. C., Hillier, L. W., Crooijmans, R. P., ... & Cheng, H. H. (2011). The development and characterization of a 60K SNP chip for chicken. BMC Genomics, 12(1), 274-283.
Hayes, B. J., Bowman, P. J., Chamberlain, A. J., & Goddard, M. E. (2009). Invited review: Genomic selection in dairy cattle: Progress and challenges. Journal of Dairy Science, 92(2), 433-443.
Henderson, C. R. (1975). Best linear unbiased estimation and prediction under a selection model. Biometrics, 31(2): 423-447.
Kennedy, J., & Eberhart, R. (1995). Particle Swarm Optimization, doi: 10.1109/icnn. 1995.488968. In Proceedings of IEEE International Conference on Neural Networks (Vol. 4, No. 1, pp. 1942-1948).
Ma, W., Qiu, Z., Song, J., Li, J., Cheng, Q., Zhai, J., & Ma, C. (2018). A deep convolutional neural network approach for predicting phenotypes from genotypes. Plant, 248(5), 1307-1318.
Md. Mohaiminul Islam (2017). Deep Learning Models for Predicting Phenotypic Traits and Diseases from Omics Data. MSc Thesis, The University of Manitoba, Canada.
Meuwissen, T.H.E., Hayes, B.J. and Goddard, M.E. (2001). Prediction of total genetic value using genome-wide dense marker maps. Genetics; 157(4):1819-1829
Okut, H., Wu, X.L., Rosa, G.J., Bauck, S., Woodward B.W., Schnabel, R.D., Taylor, J.F., and Gianola, D., (2013). Predicting expected progeny difference for marbling score in Angus cattle using artificial neural networks and Bayesian regression models. Genetics Selection Evolution, 45:34
R Core Team (2017). R: A Language and Environment for Statistical Computing. Vienna: R foundation for statistical computing.
Singh, R., Lanchantin, J., Robins, G., (2016). Deep Chrome: deep-learning for predicting gene expression from histone modifications. Bioinformatics, 32(17), 639-648.
Wenlong, M., Zhixu, Q., Jie, S., Qian, C., Chuang, M., (2017). Deep GS: Predicting phenotypes from genotypes using Deep Learning.
Yuan, Y., Peng, D., Gu, X., Gong, Y., Sheng, Z., & Hu, X. (2018). Polygenic Basis and Variable Genetic Architectures Contribute to the Complex Nature of Body Weight—A Genome-Wide Study in Four Chinese Indigenous Chicken Breeds. Frontiers in genetics, 9, 229.
Refbacks
- There are currently no refbacks.
License URL: https://www.serek.or.ke
SEREK publication https://serek.or.ke
This work is licensed under a Creative Commons Attribution 4.0 International License